iota2 classification
Assuming iota2
is fully installed, this chapter presents the main usage of iota2
: the production of a land cover map using satellite images time series.
Introduction to data
iota2
handles several sensors images :
Landsat 5 and 8 (old and new THEIA format)
Sentinel 1, Sentinel 2 L2A(THEIA and Sen2cor), Sentinel L3A (Theia Format)
Various other images already processed, with the
userFeat
sensor
In this chapter, only the use of Sentinel 2 L2A will be illustrated. To use other sensor, it is necessary to adapt the inputs parameters according to parameters descriptions.
iota2
uses machine learning algorithm to produce land cover map. It requires, among others inputs, images and related reference data”
Get the data-set
Two data-set are available, containing minimal data required to run Iota2 builders:
A entire Sentinel 2 tile, with two dates (8.8 Go)
An extraction of Sentinel 2 data, with three dates over different eco-climatic region (Soon)
The archive contains:
- /XXXX/IOTA2_TEST_S2
- archive content
content of the tutorial archive after content extraction
- ! external_code
- python code folder
user custom python code used for external features / feature maps
- external_code.py
- python code file
contains user python code used to produce the spectral indices. Rules for creating user code are explained in external features page.
- ! IOTA2_Outputs
- output folder
folder used for iota2 output folders
(empty)
- sensor_data
- input raster data
the directory which contains Sentinel-2 data. These data must be stored by tiles as in the archive.
- T31TCJ
- ! SENTINEL2A_20180511-105804-037_L2A_T31TCJ_D_V1-7
- MASKS
SENTINEL2A_20180511-105804-037_L2A_T31TCJ_D_V1-7_*.tif
SENTINEL2A_20180511-105804-037_L2A_T31TCJ_D_V1-7_FRE_B*.tif
SENTINEL2A_20180511-105804-037_L2A_T31TCJ_D_V1-7_FRE_STACK.tif
- ! SENTINEL2A_20180521-105702-711_L2A_T31TCJ_D_V1-7
- MASKS
SENTINEL2A_20180521-105702-711_L2A_T31TCJ_D_V1-7_*.tif
SENTINEL2A_20180521-105702-711_L2A_T31TCJ_D_V1-7_FRE_B*.tif
SENTINEL2A_20180521-105702-711_L2A_T31TCJ_D_V1-7_FRE_STACK.tif
- T31TDJ
- ! SENTINEL2A_20180511-105804-037_L2A_T31TDJ_D_V1-7
- MASKS
SENTINEL2A_20180511-105804-037_L2A_T31TDJ_D_V1-7_*.tif
SENTINEL2A_20180511-105804-037_L2A_T31TDJ_D_V1-7_ATB_R1.tif
SENTINEL2A_20180511-105804-037_L2A_T31TDJ_D_V1-7_FRE_B*.tif
SENTINEL2A_20180511-105804-037_L2A_T31TDJ_D_V1-7_FRE_STACK.tif
SENTINEL2A_20180511-105804-037_L2A_T31TDJ_D_V1-7_MTD_ALL.xml
SENTINEL2A_20180511-105804-037_L2A_T31TDJ_D_V1-7_QKL_ALL.jpg
- ! SENTINEL2A_20180521-105702-711_L2A_T31TDJ_D_V1-7
- MASKS
SENTINEL2A_20180521-105702-711_L2A_T31TDJ_D_V1-7_*.tif
SENTINEL2A_20180521-105702-711_L2A_T31TDJ_D_V1-7_ATB_R1.tif
SENTINEL2A_20180521-105702-711_L2A_T31TDJ_D_V1-7_FRE_B*.tif
SENTINEL2A_20180521-105702-711_L2A_T31TDJ_D_V1-7_FRE_STACK.tif
SENTINEL2A_20180521-105702-711_L2A_T31TDJ_D_V1-7_MTD_ALL.xml
SENTINEL2A_20180521-105702-711_L2A_T31TDJ_D_V1-7_QKL_ALL.jpg
- vector_data
- input vector data
directory containing input vector data
reference_data.cpg
reference_data.dbf
reference_data.prj
- reference_data.shp
- shapefile
the shapeFile containing geo-referenced and labelled polygons (no multi-polygons, no overlapping) used to train a classifier.
reference_data.shx
EcoRegion.dbf
EcoRegion.prj
EcoRegion.qpj
- EcoRegion.shp
- shapefile
shapeFile containing two geo-referenced polygons representing a spatial stratification (eco-climatic areas, for instance).
EcoRegion.shx
- colorFile.txt
- color table
colors used in classification map
$ cat colorFile.txt ... 211 255 85 0 ...
Here the class 211 has the RGB color 255 85 0.
- IOTA2_Example.cfg
- example config file
the file used to set iota2’s parameters such as inputs/outputs paths, classifier parameters etc.
- i2_tutorial_classification.cfg
- sample config file
sample config file for classification builder
- i2_tutorial_features_map.cfg
- sample config file
sample config file for feature map builder
- i2_tutorial_obia.cfg
- sample config file
sample config file for object base image analysis
- nomenclature23.txt
- nomenclature file
label’s name. The purpose of the file is to get a pretty results report at the end of the chain by relabeling integers labels by a more verbose type.
& cat nomenclature.txt ... prairie:211 ...
Here the class 211 corresponds to the class prairie
vecteur_23.qml
Warning
Each class must be represented in colorFile.txt and nomenclature.txt
Understand the configuration file
iota2
is configured through several of parameters, some of them are specfic to iota2
and some belong to other libraries such as scikit-learn
or OTB
.
These parameters allow to select the operations to be carried out and their various parameters. A documentation of all these parameters is provided here. The user defines these paramereters in a configuration file (a human readable text file) that is read by iota2
at upon start. The file is structured into sections, each section containing several fields.
To simplify the use, iota2
read a configuration file which is a simple text file containing sections and fields. A section (or group of fields) contains fields with similar purposes, for instance the section chain contain general information such as input data, and the output path and the section arg_train will contains informations about the classifier’s parameters.
The minimal configuration file contains all required fields to produce a land cover map.
chain :
{
output_path : '/XXXX/IOTA2_TEST_S2/IOTA2_Outputs/Results'
remove_output_path : True
nomenclature_path : '/XXXX/IOTA2_TEST_S2/nomenclature.txt'
list_tile : 'T31TCJ'
s2_path : '/XXXX/IOTA2_TEST_S2/sensor_data'
ground_truth : '/XXXX/IOTA2_TEST_S2/vector_data/groundTruth.shp'
data_field : 'code'
spatial_resolution : 10
color_table : '/XXXX/IOTA2_TEST_S2/colorFile.txt'
proj : 'EPSG:2154'
first_step:"init"
last_step: "validation"
}
arg_train :
{
classifier : 'rf'
otb_classifier_options : {'classifier.rf.min': 5,'classifier.rf.max': 25 }
}
arg_classification :
{
classif_mode : 'separate'
}
task_retry_limits:
{
allowed_retry : 0
maximum_ram : 60.0
maximum_cpu : 12
}
For an end user, launching iota2
requires to fill correctly the configuration file.
In the above example, replace the XXXX
by the path where the archive has been extracted.
Running the chain
iota2 launch
The chain is launched with the following command line.
Iota2.py -config /XXXX/IOTA2_TESTS_DATA/config_tuto_classif.cfg -scheduler_type localCluster
First, the chain displays the list of all steps activated by the configuration file
Group init: [x] Step 1: check inputs [x] Step 2: Sensors pre-processing [x] Step 3: Generate a common masks for each sensors [x] Step 4: Compute validity raster by tile Group sampling: [x] Step 5: Generate tile's envelope [x] Step 6: Generate a region vector [x] Step 7: Prepare samples [x] Step 8: merge samples by models [x] Step 9: Generate samples statistics by models [x] Step 10: Select pixels in learning polygons by models [x] Step 11: Split pixels selected to learn models by tiles [x] Step 12: Extract pixels values by tiles [x] Step 13: Merge samples dedicated to the same model Group learning: [x] Step 14: Learn model Group classification: [x] Step 15: Generate classifications Group mosaic: [x] Step 16: Mosaic Group validation: [x] Step 17: generate confusion matrix by tiles [x] Step 18: Merge all confusions [x] Step 19: Generate final report
Once the processing start, a large amount of information will be printed, most of them concerning the dask-scheduler.
Did it all go well?
iota2
is packed with a logging system. Each step has its has its own log folder, available in the output_path/logs
directory (see logs in Output tree structure)
In these directories two kind of log can be found *_out.log
and *_err.log
.
The error are compiled in “err” file and the standard output in “out” file.
With the scheduler dask, iota2
go as far as possible while the data required for the next steps is available.
To simplify the error identification, an interactive graph is produced in a html page.
To open it, open the index.html
file in html
folder.
Nodes in graph can have three colors (red: error, blue: done, orange: not yielded).
By clicking on graph node, the corresponding log file is openned.
If despite all this information, the errors can not be identifiyed or solved, the iota2
team can help all users.
The simplest way to ask help is to create an issue on framagit by adding the archive available in log directory.
Output tree structure
In this section, the iota2
outputs available after a proper run are described.
- /XXXX/IOTA2_TEST_S2/IOTA2_Outputs/Results
- output folder
output folder defined in config file output_path
- ! classif
- per tile classification maps
- Contains classification maps, for each tile and each region. They will be merged in the
final
directory.
Classif_T31TCJ_model_1_seed_0.tif
- ! MASK
MASK_region_1_T31TCJ.tif
T31TCJ_model_1_confidence_seed_0.tif
tmpClassif
- ! config_model
empty
(empty)
- ! dataAppVal
- desc
- Shapefiles obtained after spliting reference data between learning and validation set according a ratio.
- ! bymodels
(empty)
T31TCJ_seed_0_learn.sqlite
T31TCJ_seed_0_val.sqlite
- ! dataRegion
- vector data split by region
- When using eco-climatic region, contains the vector data split by region.
(empty)
- ! envelope
- shapefiles
- Contains shapefiles, one for each tile.Used to ensure tile priority, with no overlap.
T31TCJ.dbf
T31TCJ.prj
T31TCJ.shp
T31TCJ.shx
- ! features
- useful information
- for each tile, contains useful information
- T31TCJ
- ! tmp
- temporary folder
- folder created temporarily during the chain execution
MaskCommunSL.dbf
MaskCommunSL.prj
- MaskCommunSL.shp
- common scene
- the common scene of all sensors for this tile.
MaskCommunSL.shx
MaskCommunSL.tif
- Sentinel2L3A_T31TCJ_reference.tif
- reference image
- the image, generated by
iota2
, used for reprojecting data
- Sentinel2L3A_T31TCJ_input_dates.txt
- list of dates
- the list of date detected in
s2_path
for the current tile.
Sentinel2_T31TCJ_interpolation_dates.txt
CloudThreshold_0.dbf
CloudThreshold_0.prj
- CloudThreshold_0.shp
- database used as mask
- This database is used to mask training polygons according to a number of clear date. See cloud_threshold parameter
CloudThreshold_0.shx
- nbView.tif
- number visits
- number of time a pixel is seen in the whole time series (i.e., excluding clouds, shadows, staturation and no-data)
- final
- final producs
- This folder contains the final products of
iota2
.All final products will be generated in thefinal
directorysee Final products for details
- ! simplification
mosaic
tiles
tmp
vectors
- ! TMP
ClassificationResults_seed_0.txt
Classif_Seed_0.csv
T31TCJ_Cloud.tif
T31TCJ_GlobalConfidence_seed_0.tif
T31TCJ_seed_0_CompRef.tif
T31TCJ_seed_0.csv
T31TCJ_seed_0.tif
Classif_Seed_0_ColorIndexed.tif
Classif_Seed_0.tif
Confidence_Seed_0.tif
Confusion_Matrix_Classif_Seed_0.png
diff_seed_0.tif
PixelsValidity.tif
RESULTS.txt
vectors
- ! formattingVectors
- learning samples
- The learning samples contained in each tiles.Shapefiles in which pixel values from time series have been extracted.
- ! T31TCJ
- temporary directory
- This is a temporary working directory, intermediate files are (re)moved after step completion.
(empty)
T31TCJ.cpg
T31TCJ.dbf
T31TCJ.prj
T31TCJ.shp
T31TCJ.shx
- ! learningSamples
- learning samples
- Sqlite file containing learning samples by regions.Also contains a CSV file containing statistics about samples balance for each seed. See tracing back samples to generate this file manually.
class_statistics_seed0_learn.csv
Samples_region_1_seed0_learn.sqlite
T31TCJ_region_1_seed0_Samples_learn.sqlite
- ! logs
- logs
- output logs of
iota2
. See Did it all go well? section for details
- classification
classification_T31TCJ_model_1_seed_0.err
classification_T31TCJ_model_1_seed_0.out
- CommonMasks
common_mask_T31TCJ.err
common_mask_T31TCJ.out
- confusionCmd
confusion_T31TCJ_seed_0.err
confusion_T31TCJ_seed_0.out
- confusionsMerge
merge_confusions.err
merge_confusions.out
- Envelope
tiles_envelopes.err
tiles_envelopes.out
- genRegionVector
region_generation.err
region_generation.out
- ! html
configuration_file.html
environment_info.html
genindex.html
index.html
input_files_content.html
objects.inv
output_path_content.html
s2_path_content.html
search.html
searchindex.js
- source
classification_T31TCJ_model_1_seed_0.out
common_mask_T31TCJ.out
configuration_file.rst
confusion_T31TCJ_seed_0.out
environment_info.rst
extraction_T31TCJ.out
final_report.out
index.rst
input_files_content.rst
learning_model_1_seed_0.out
merge_confusions.out
merge_model_1_seed_0_usually.out
merge_samples_T31TCJ.out
mosaic.out
output_path_content.rst
preprocessing_T31TCJ.out
region_generation.out
s2_path_content.rst
s_sel_model_1_seed_0.out
stats_1_S_0_T_T31TCJ.out
tasks_status_1.rst
tasks_status_2.rst
tiles_envelopes.out
validity_raster_T31TCJ.out
vector_form_T31TCJ.out
- _sources
configuration_file.rst.txt
environment_info.rst.txt
index.rst.txt
input_files_content.rst.txt
output_path_content.rst.txt
s2_path_content.rst.txt
tasks_status_1.rst.txt
tasks_status_2.rst.txt
- _static
basic.css
- css
badge_only.css
- fonts
fontawesome-webfont.eot
fontawesome-webfont.svg
fontawesome-webfont.ttf
fontawesome-webfont.woff
fontawesome-webfont.woff2
lato-bold-italic.woff
lato-bold-italic.woff2
lato-bold.woff
lato-bold.woff2
lato-normal-italic.woff
lato-normal-italic.woff2
lato-normal.woff
lato-normal.woff2
Roboto-Slab-Bold.woff
Roboto-Slab-Bold.woff2
Roboto-Slab-Regular.woff
Roboto-Slab-Regular.woff2
theme.css
doctools.js
documentation_options.js
file.png
jquery-3.5.1.js
jquery.js
- js
badge_only.js
html5shiv.min.js
html5shiv-printshiv.min.js
theme.js
language_data.js
minus.png
plus.png
pygments.css
searchtools.js
underscore-1.3.1.js
underscore.js
- tasks_status_1.html
tasks_status_2.html
- learnModel
learning_model_1_seed_0.err
learning_model_1_seed_0.out
- mosaic
mosaic.err
mosaic.out
- PixelValidity
validity_raster_T31TCJ.err
validity_raster_T31TCJ.out
- reportGeneration
final_report.err
final_report.out
- samplesByModels
merge_model_1_seed_0_usually.err
merge_model_1_seed_0_usually.out
- samplesByTiles
merge_samples_T31TCJ.err
merge_samples_T31TCJ.out
- samplesExtraction
extraction_T31TCJ.err
extraction_T31TCJ.out
- samplesMerge
merge_model_1_seed_0.err
merge_model_1_seed_0.out
- samplingLearningPolygons
s_sel_model_1_seed_0.err
s_sel_model_1_seed_0.out
- sensorsPreprocess
preprocessing_T31TCJ.err
preprocessing_T31TCJ.out
- statsSamplesModel
stats_1_S_0_T_T31TCJ.err
stats_1_S_0_T_T31TCJ.out
tasks_status_1.svg
tasks_status_2.svg
- VectorFormatting
vector_form_T31TCJ.err
vector_form_T31TCJ.out
- ! model
- desc
- The learned models
model_1_seed_0.txt
- ! samplesSelection
- shapefiles
- Shapefiles containing points (or pixels coordinates) selected for training stage.Also contains a CSV summary of the actual number of samples per class
samples_region_1_seed_0.dbf
samples_region_1_seed_0_outrates.csv
samples_region_1_seed_0.prj
samples_region_1_seed_0_selection.sqlite
samples_region_1_seed_0.shp
samples_region_1_seed_0.shx
samples_region_1_seed_0.xml
T31TCJ_region_1_seed_0_stats.xml
T31TCJ_samples_region_1_seed_0_selection.sqlite
T31TCJ_selection_merge.sqlite
- ! shapeRegion
- desc
- Shapefiles indicating intersection between tiles and region.
MyRegion_region_1_T31TCJ.dbf
MyRegion_region_1_T31TCJ.prj
MyRegion_region_1_T31TCJ.shp
MyRegion_region_1_T31TCJ.shx
MyRegion_region_1_T31TCJ.tif
- ! stats
- statistics
- Optional xml statistics to standardize the data before learning (svm…).
(empty)
- IOTA2_tasks_status.txt
- internal execution status
iota2
keeps track of it’s execution using this pickle file (not text) to be allowed to restart from the state where it stopped.
- logs.zip
logs archive
MyRegion.dbf
MyRegion.prj
- MyRegion.shp
- fake region
- When no ecoclimatic region is defined for learning step,
iota2
creates this fake file with a single region.
MyRegion.shx
reference_data.dbf
reference_data.prj
- reference_data.shp
- reencoded shapefile
- As OTB expects classes to be encoded as consecutive integers, which is not necessarily the case of user labels, this shapefile contains user data with reencoded labels.
reference_data.shx
Final products
All final products will be generated in the final
directory
Land cover map
Your Classif_Seed_0_ColorIndexed.tif should look like this one:
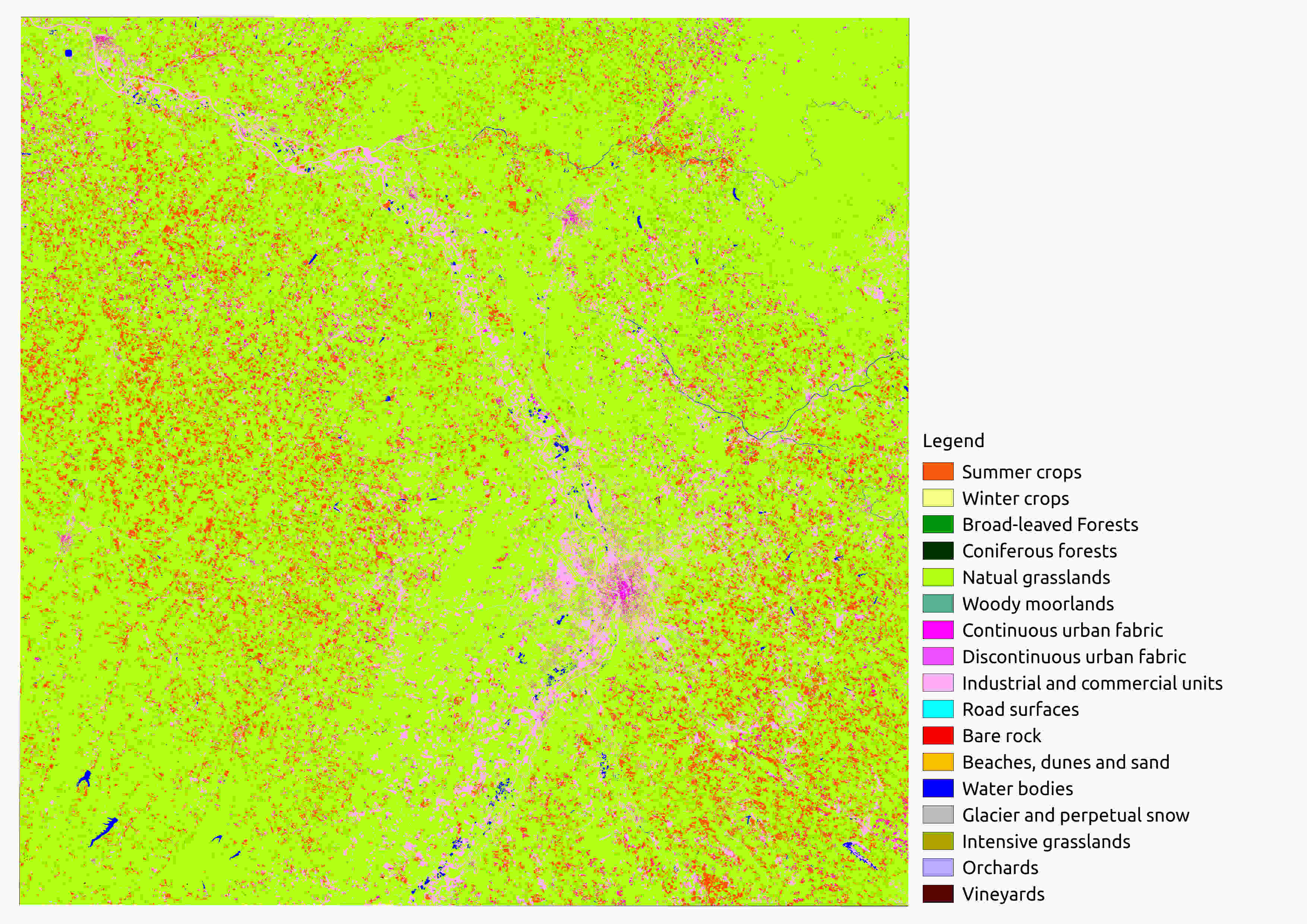
Classif_Seed_0.tif Example
This map contains labels from the shapeFile groundTruth.shp
. As you can see the classification’s quality is rather low.
A possible explanation is the low number of dates used to produce it. A raster called PixelsValidity.tif
gives the number of dates for which the pixel is clear (no cloud, cloud shadow, saturation)
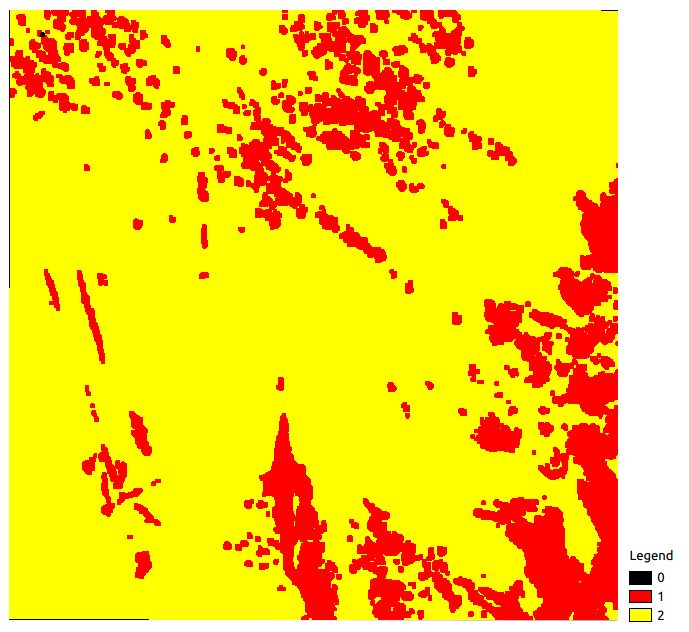
PixelsValidity.tif Example
As only two dates are used to produce the classification map, pixels are in the [0; 2] range. iota2
also provides a confidence map: Confidence_Seed_0.tif
which
allows to better understand the resulting classification. This map gives for each pixel a scale between O and 100, where 0 and 100 meant that the probability membership provided by the classifier is 0 and 1, respectively. This is not a validation, just an estimate of the confidence in the decision of the classifier.
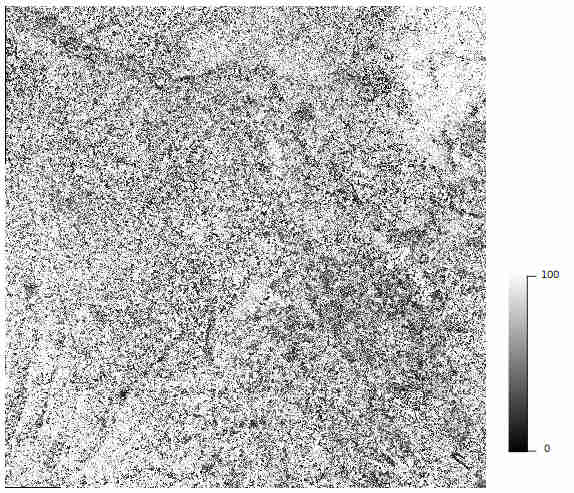
Confidence_Seed_0.tif Example
These three maps form iota2
’s main outputs: they are the minimum outputs required to analyse and understand the results.
We analyzed and produced classifications thanks to iota2
. The main objective is to get the better land cover map as possible. There are many ways to achieve this purpose: researchers publish every day new methods.
The simplest method to get better results can consist in using a longer time series, improving the reference data for training, etc.
Measuring quality
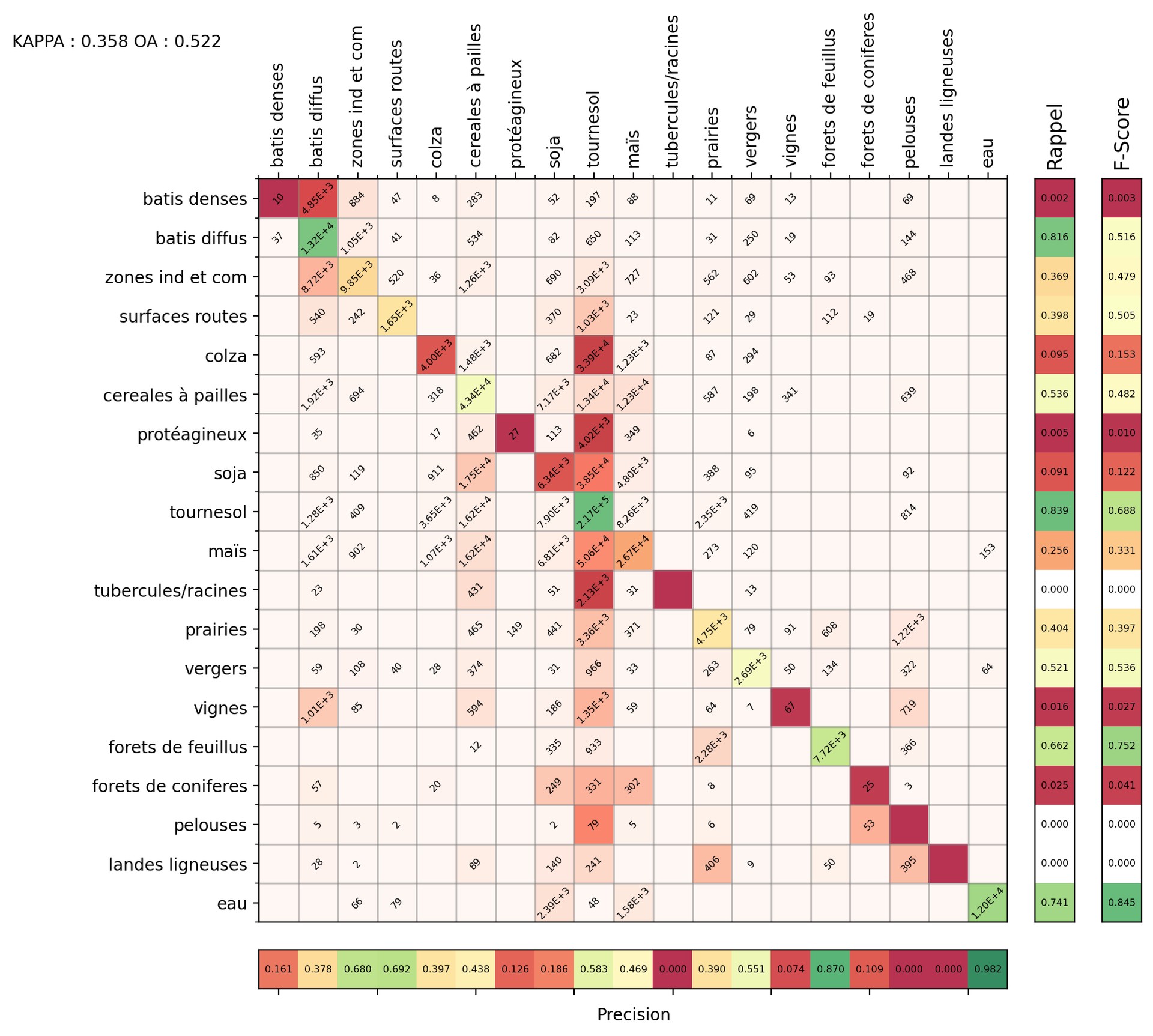
Confusion_Matrix_Classif_Seed_0.png Example
Confusion matrices allow us to measure the quality of a classification. In the one provided by iota2
(in the final
output directory), the pixels whose labels are known (the reference) are in rows and the inferred pixels are in columns.